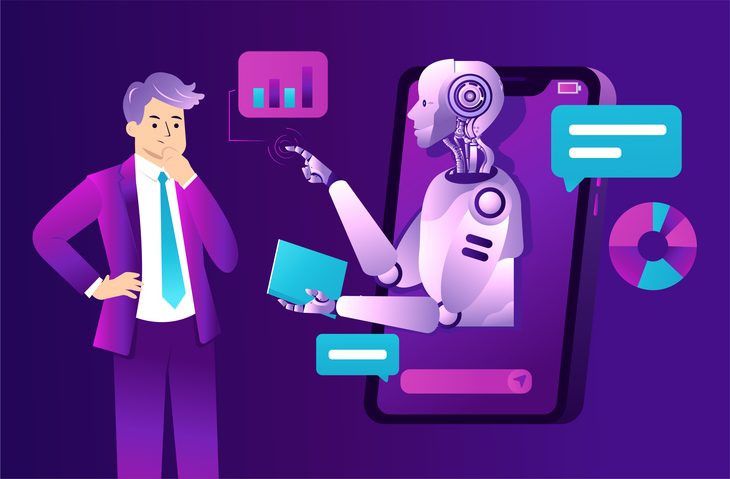
Personalization, trust, and adaptability: Key challenges for AI usability
As AI-powered systems become increasingly central to our digital experiences, the way we approach usability testing needs to evolve. AI interfaces are dynamic, adapting and learning from user interactions, which means traditional testing methods often fall short. To create products that users trust and enjoy, we need to develop new strategies for usability testing for AI interfaces — strategies that account for the complexity, adaptability, and personalization inherent in these systems.
Now, let me take you to a personal example to show what I mean.
My Spotify story: Personalization at its best
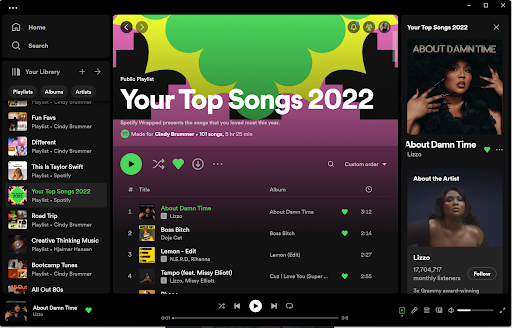
I’m a Spotify junkie. I use it all the time. Anyone else out there a fan? If you’ve spent even five minutes on the app, you know how deeply it personalizes your experience. My Spotify dashboard is tailored to my tastes—music I love, podcasts I might enjoy, and playlists designed just for me. One of my favorite features is the yearly “Top Songs” playlist. Here’s a look at my 2022 list (warning: it’s eclectic).
This year, Spotify also rolled out a feature called Smart Shuffle, which suggests new songs to add to my playlists based on my listening habits. It’s a great example of how AI uses algorithms to create a highly personalized user experience.
But here’s the thing: that personalization isn’t static. It changes constantly based on my interactions. And this brings me to the challenge of usability testing for AI interfaces — traditional methods just aren’t enough to evaluate systems that adapt and evolve over time.
Why AI interfaces are different
AI systems like Spotify rely on algorithms to drive their decision-making. These algorithms “learn” from user behavior, creating a dynamic and personalized experience. While this makes AI incredibly powerful, it also poses unique challenges for usability testing.
Here’s why traditional usability testing methods don’t always work for AI interfaces:
1. Static vs. dynamic experiences
Traditional testing assumes a static interface — one that looks and behaves the same for every user. But AI interfaces are dynamic, changing based on user preferences and behaviors.
2. Short-term vs. long-term interactions
Most usability tests are short-term, focusing on immediate user interactions. AI systems, however, adapt over time, meaning their usability needs to be assessed over weeks, months, or even years.
3. Visual vs. behavioral focus
Traditional tests focus on visible elements like navigation and clicks. With AI, the interactions often involve text responses, recommendations, or predictions, which require a deeper behavioral analysis.
4. Universal vs. personalized experiences
Traditional testing methods evaluate interfaces designed for universal use. AI interfaces, by contrast, are highly personalized, requiring a more nuanced approach to testing.
What makes usability testing for AI interfaces unique?
When it comes to testing AI systems, the approach needs to reflect the unique challenges and opportunities these interfaces present. Unlike traditional static systems, AI interfaces are dynamic, constantly learning and adapting to user behavior. This creates a complex relationship between the user and the AI that requires a deeper level of understanding during usability testing.
Here are the key areas to explore when evaluating the usability of AI systems:
Understanding and trusting AI’s decision-making
AI systems make decisions based on algorithms that often feel opaque to users. One of the first challenges in usability testing is assessing whether users comprehend how the AI reaches its conclusions. Do they feel the system is transparent enough? Or are they left guessing why a recommendation or prediction was made? A lack of clarity can erode trust and ultimately deter users from engaging with the product.
For example, if Spotify recommends a song that feels completely out of place, users might wonder, “Why did it think I’d like this?” Testing should uncover these moments of confusion and assess whether clearer explanations—like showing “based on your recent listens” prompts—could enhance understanding.
Perception of AI-generated outputs
AI systems often provide outputs such as recommendations, predictions, or even warnings. The effectiveness of these outputs depends not just on their accuracy but on how users perceive their value. Are the recommendations helpful? Do users feel the predictions are reliable? It’s not enough for the system to be “technically correct”—users must see the outputs as meaningful and actionable.
Consider a healthcare app that predicts potential risks based on patient data. Even if the prediction is accurate, if it’s presented without context or actionable next steps, users may feel frustrated or distrustful. Testing must evaluate how users interpret these outputs and whether the interface supports their needs.
The evolution of trust over time
Trust is a cornerstone of any successful AI interface, but it’s not a static quality—it evolves over time. Users may start with skepticism but grow to trust the system as they see consistent, reliable results. Conversely, a single major error can undermine trust built over weeks or months.
Usability testing for AI interfaces should include long-term studies to assess how trust develops. Do users begin to rely on the system more after repeated positive experiences? Or do errors—no matter how infrequent—erode their confidence? Understanding this evolution is critical to improving user retention and satisfaction.
Recovery from errors or unexpected situations
No system is perfect, and AI is no exception. What happens when the AI gets something wrong or encounters a scenario it wasn’t designed to handle? Usability testing should evaluate not only the frequency of these errors but also how well users can recover from them.
For instance, if a navigation app gives a user an incorrect route, can they easily correct it? Or does the error leave them stranded, unsure how to proceed? Testing must explore whether users can identify and correct errors without feeling frustrated or abandoned.
Adapting to individual preferences
Personalization is a hallmark of AI interfaces, but how well does the system actually tailor itself to the user? More importantly, do users notice and appreciate these adaptations? Usability testing should assess whether the AI’s adjustments feel intuitive and aligned with user preferences—or whether they create unexpected friction.
For example, Spotify’s ability to adapt to my eclectic music taste is great—until it starts including recommendations based on my kids’ preferences. Testing can reveal whether users feel the system “understands” them or whether they’re frustrated by its missteps.
The role of specialized techniques
Addressing these challenges requires specialized techniques that go beyond traditional usability testing methods. Techniques like adaptive testing, sentiment analysis, and long-term studies provide the insights needed to evaluate the unique characteristics of AI interfaces. These approaches allow us to measure not just immediate usability but the ongoing relationship between the user and the AI.
Let’s dive into three of these specialized techniques to see how they can help refine AI interfaces and improve the user experience.
Three techniques for usability testing for AI interfaces
1. Adaptive testing
AI interfaces change their behavior based on user interactions, so our testing methods need to adapt as well. This is where adaptive testing comes in.
Instead of testing with a single, homogeneous user group, adaptive testing involves working with a diverse set of user profiles. Think beyond demographics and consider behavioral traits, technical proficiency, or unique use cases. For example:
- Testing Spotify with users who prefer different genres (pop, classical, hip-hop).
- Including users who primarily listen in specific settings, like while driving or working out.
- Recruiting parents who share their accounts with kids (as someone with kids, my Spotify recommendations are… interesting).
By observing these diverse profiles, we can evaluate whether the AI effectively adapts to each user’s needs and identify where it might fall short. This is a critical component of usability testing for AI interfaces, as it highlights how well the system personalizes the experience.
2. Emotion and sentiment analysis
AI interfaces don’t just affect users’ actions—they affect their emotions. An essential part of usability testing for AI interfaces is understanding how users feel during their interactions.
Here’s how emotion and sentiment analysis works:
- Collect user-generated data. This can include chat logs, text inputs, or speech recordings.
- Analyze emotional cues. Look for words, phrases, or tones that indicate positive, negative, or neutral feelings.
- Classify sentiments. Use tools (or manual methods) to determine whether users feel frustrated, delighted, or indifferent.
By analyzing these emotional responses, we can identify pain points and areas where the AI interface builds—or breaks—trust.
3. Long-term testing
AI systems evolve as they learn from user interactions. To truly understand their usability, we need to take a long-term view through longitudinal testing.
Longitudinal studies track the same participants over an extended period, assessing how their perceptions and interactions change. For example:
- Initial test: How intuitive is the AI during the first interaction?
- Follow-up test: Does the user find the AI’s recommendations more accurate after a month?
- Final test: After several months, does the user trust the AI more or less?
When conducting long-term studies, remember:
- Set clear expectations about the time commitment upfront.
- Offer additional incentives to keep participants engaged.
- Use consistent metrics to track changes over time.
Long-term testing is invaluable for usability testing for AI interfaces, as it reveals how trust and satisfaction evolve alongside the AI’s learning process.
Building trust and transparency in AI interfaces
Trust and transparency are the foundation of a great AI interface. Users need to understand why the AI makes certain decisions—and feel confident that those decisions are in their best interest.
Take Spotify’s Smart Shuffle feature. When it recommends a great song, I’m thrilled. But when it misfires (country music on my rock playlist?), my trust in the system takes a hit. This underscores the importance of usability testing in evaluating how transparent and trustworthy an AI interface feels to users.
Conclusion: Adapt your usability testing methods
The world of AI interfaces is dynamic, adaptive, and deeply personalized. To ensure these systems are truly usable, we need to go beyond traditional testing methods. By incorporating adaptive testing, sentiment analysis, and long-term studies, we can gain deeper insights into user experiences and create interfaces that inspire trust and delight.
Remember, usability testing for AI interfaces isn’t just about evaluating clicks or navigation—it’s about understanding the complex, evolving relationship between humans and machines. And that’s what makes this work so exciting.
Happy testing!