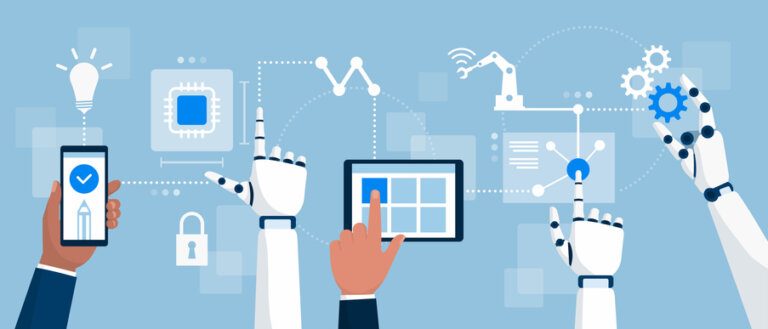
How UX patterns differ when you’re building AI applications
When you’re building an AI application, you need the right tools for the job. Just a construction team needs different materials and tools for building a skyscraper than they would for a house, an AI application needs UX patterns that meet its needs.
While traditional UX focuses on predictable and linear interactions, AI-driven UX introduces a layer of complexity and adaptability that demands a fresh approach.
In this article, I’ll explore the key differences between AI-driven UX patterns and standard UX patterns and highlight areas designers should consider to stay ahead.
Eight AI-driven UX patterns redefining user experience
1. Predictive vs. deterministic behavior
Standard interfaces are built around predictable interactions. For example, clicking a “Submit” button on a form reliably triggers form submission, leading to a consistent outcome every time.
AI-driven UX shifts the interface from fixed responses to adaptive, prediction-based interactions, which ultimately changes how users engage with interfaces.
Understanding the difference between predictive and deterministic behavior is important because AI-driven systems introduce variability and adaptability into user interactions, which traditional UX designs typically do not account for.
AI/ML-Based UX: AI-driven systems utilize statistical models that can produce varying outcomes based on different inputs.
Take Netflix’s recommendation engine, which suggests content based on viewing history and can change recommendations as it learns more about user preferences. This variability means that the designs have to communicate uncertainty and provide explanations for dynamic results.
The AI-driven UX pattern: Adaptive Response Systems
Adaptive Response Systems leverage AI to predict user needs and adjust interactions dynamically. Instead of offering a one-size-fits-all response, the system anticipates various user actions and tailors outcomes based on contextual data and user behavior patterns.
2. Transparency & explainability
Users expect clear and consistent outcomes. For example, a disabled button typically means a form isn’t complete, and users can easily understand why an action isn’t available. But it needs to go further with AI applications. Users want to understand how AI-driven decisions are made.
AI/ML-Based UX: When AI influences decisions, users need to understand why certain jobs are suggested. Studies have shown that 82 percent of consumers believe that AI requires human oversight to be trusted (IBM), and 84 percent are more likely to engage with companies that clearly explain how they use AI (Accenture). Designers must incorporate explainable elements.
For example, LinkedIn’s job recommendation system includes explanations such as “Recommended based on your skills and past job searches,” to help users understand the rationale behind each suggestion.
The AI-driven UX pattern: Explainable AI Interfaces
Explainable AI Interfaces integrate elements that make AI decisions transparent to users. This includes providing clear explanations for recommendations, predictions, or actions taken by the AI, fostering trust and understanding.
3. Real-time adaptation & personalization
In traditional UX, personalization often involves user-selected preferences, like choosing a theme color or setting notification preferences manually. It doesn’t need to change.
But for AI applications, real-time adaptation and personalization are essential because they allow AI-driven interfaces to dynamically respond to individual user behaviors and preferences. This not only enhances user satisfaction but also drives higher levels of interaction and loyalty, as users feel that the application truly understands and meets their unique needs.
AI/ML-Based UX: AI can autonomously adapt to user behaviors in real-time. For example, Google Photos automatically categorizes and suggests albums based on user activity without manual input. Designers must implement safeguards, such as easy options to undo or customize AI suggestions, ensuring that personalization feels seamless and not intrusive.
The AI-driven UX pattern: Dynamic Personalization Engines
Dynamic Personalization Engines continuously analyze user behavior and context to adjust the interface and content in real-time. This ensures that the user experience remains relevant and engaging without requiring manual input from the user.
4. Expanded error states & “in-between” states
In traditional UX, error handling is straightforward, dealing with clear-cut issues like broken links or incorrect form entries. States are clear cut and fixed.
But it’s not so clear cut in AI applications. AI-driven systems often encounter uncertainties or partial results that traditional UX designs don’t address.
AI/ML-Based UX: AI introduces nuanced error states. For instance, a voice assistant like Amazon’s Alexa might misinterpret a command, leading to partial or irrelevant responses. Designers need to create messaging that addresses these gray areas, offering users ways to clarify or correct AI misunderstandings.
Effectively managing these nuanced states ensures a smoother user experience, minimizes frustration, and maintains user trust even when the AI cannot provide definitive answers.
The AI-driven UX pattern: Graceful Degradation Messaging
Graceful Degradation Messaging handles AI uncertainties and partial failures by providing nuanced feedback to users. Instead of binary error messages, the system offers suggestions, clarifications, or alternative options when AI predictions are uncertain or incomplete.
For example, Amazon’s Alexa might respond with, “I’m not sure I understood that. Did you mean [Option A] or [Option B]?” when a voice command is ambiguous, allowing users to clarify their intent.
5. Continuous feedback loops
In standard applications, feedback is typically gathered through periodic user testing or analytics, leading to updates based on aggregated data. But AI systems are constantly gathering feedback.
AI/ML-Based UX: AI systems thrive on continuous feedback. For example, Grammarly refines its suggestions based on real-time user corrections and confirmations. Integrating these “teach the system” loops into the design ensures that the product evolves with user input, enhancing accuracy and relevance over time.
Continuous feedback loops are vital because they enable AI-driven systems to learn and improve in real time based on user interactions. This ongoing adaptation ensures that the user experience remains relevant and personalized, fostering higher engagement and satisfaction by continuously aligning the product with user needs and preferences.
The AI-driven UX pattern: Interactive Learning Interfaces
Interactive Learning Interfaces incorporate mechanisms for users to provide real-time feedback, which the AI uses to improve its performance. This creates an ongoing dialogue between the user and the system, enhancing the AI’s accuracy and relevance over time.
Grammarly allows users to accept or reject its suggestions, using this feedback to refine its grammar and style recommendations based on individual writing preferences.
6. Ethical & trust considerations
Ethics and trust are always important. Traditional UX focuses on privacy policies, data security, and avoiding deceptive designs to maintain user trust.
AI/ML-Based UX goes further by addressing algorithmic biases and ensuring fairness. Ethical and trust considerations are crucial because AI-driven systems can introduce biases, impact user privacy, and make decisions that significantly affect individuals. Ensuring these systems operate transparently and fairly is essential for building and maintaining user trust and fostering responsible AI interactions.
Designers must prioritize ethical data usage, actively work to eliminate biases, and transparently communicate how user data influences AI behaviors to maintain and build trust.
The AI-driven UX pattern: Ethical Transparency Panels
Ethical Transparency Panels are dedicated sections within the interface that inform users about how their data is used, the measures taken to ensure fairness, and the steps implemented to mitigate biases. This promotes ethical accountability and builds user trust.
Going back to our above example, Facebook provides transparency reports and explanations about how content recommendation algorithms are designed to minimize bias and promote diverse content, so that users are aware of the ethical considerations in place.
7. Need for user education & onboarding
In traditional UX, onboarding typically involves tutorials or tooltips that explain a fixed set of features. For AI applications, user education and onboarding needs to go further.
AI/ML-Based UX: Users may need guidance on interacting with AI features because AI-driven features often involve complex functionalities that users may not intuitively understand. Effective onboarding should clarify the AI’s capabilities, limitations, and how users can interact with it to maximize benefits, such as “Here’s how you can help the AI get smarter by categorizing your transactions.”
And there are a lot of reasons for prioritizing education and onboarding.
- Enhanced user understanding and trust
Gartner reported that 70 percent of organizations implementing AI believe that user education is critical to fostering trust and ensuring successful adoption of AI technologies. - Improved user engagement and satisfaction
A PwC survey found that 73 percent of consumers are more likely to engage with AI-powered applications that provide clear instructions and educational resources on how to use AI features effectively. - Reduction in user frustration and abandonment
According to UserIQ, 60 percent of users are more likely to abandon an application if they find its AI features confusing or difficult to use, underscoring the need for comprehensive onboarding processes. - Increased use of AI features
Salesforce discovered that products with robust onboarding experiences see a 25 percent higher adoption rate of advanced AI functionalities compared to those with minimal or no onboarding support. - Facilitating continuous learning and adaptation
A study by McKinsey indicated that 80 percent of users appreciate ongoing education and updates about AI capabilities, which helps them adapt to new features and maximizes the utility of the application.
The AI-driven UX pattern: AI Onboarding Tutorials
AI Onboarding Tutorials are interactive guides that educate users about the AI’s capabilities, limitations, and how to effectively interact with it. These tutorials help users understand how to maximize the benefits of AI features and contribute to their improvement.
For example, Mint’s personal finance app includes onboarding steps that explain how its AI analyzes spending patterns and offers tips on categorizing transactions to help the AI provide more accurate financial insights.
8. Dynamic evolution of the experience
In traditional applications, UX design changes are deliberate and tied to version releases, allowing users to anticipate and adapt to updates. But AI is faster and more dynamic. AI-driven interfaces to continuously adapt and improve based on real-time user interactions and data
The AI/ML-Based UX can evolve autonomously as AI models improve. Designers must implement robust testing and monitoring to ensure these changes align with user expectations and enhance the overall experience.
This ongoing adaptation ensures that the user experience remains relevant and engaging, effectively meeting changing user needs and preferences without the delays associated with traditional manual updates.
The AI-driven UX pattern: Continuous Improvement Frameworks
Continuous Improvement Frameworks ensure that the user experience evolves seamlessly as AI models are updated and datasets grow. This involves rigorous testing, monitoring, and user feedback integration to maintain alignment with user needs and expectations.
For example, Spotify’s Discover Weekly playlist automatically updates based on users’ latest listening habits without requiring a new app version, ensuring the playlist remains fresh and tailored to current preferences.
The bottom line
While foundational UX principles like consistency, intuitive navigation, and user-centric design remain important, AI-powered products introduce unique challenges. Designers must navigate uncertainty, accommodate evolving behaviors, and foster deeper user feedback loops.
Success in AI-driven UX patterns hinges on prioritizing transparency, explainability, controllable personalization, and ethical responsibility — elements that extend beyond traditional, static user interface design.
Additional considerations
While the above points cover significant differences between AI-driven UX patterns and traditional UX patterns, it’s also essential to consider:
- Scalability: As AI systems handle more complex tasks, ensuring that the UX scales without becoming overwhelming is crucial.
- Cross-platform consistency: Maintaining a consistent experience across various devices and platforms becomes more challenging with AI’s dynamic nature.
- Accessibility: AI can both enhance and hinder accessibility. Designers must ensure that AI features are inclusive, providing support for users with diverse needs.
By integrating these AI-driven UX patterns into your design strategy, you can create more intuitive, responsive, and trustworthy user experiences that leverage the full potential of artificial intelligence while addressing its inherent complexities.