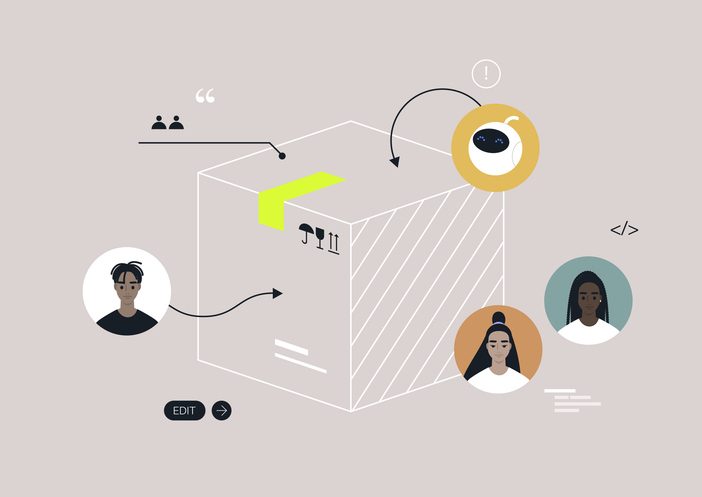
The steps to add AI features follow a similar, but slightly different core process
I’m going to start this article on how to implement AI in SaaS products with an unexpected example. Do you remember Tickle Me Elmo?
In 1996, the “Tickle Me Elmo” doll was the hottest toy of the season. It was so hot, that parents were buying out stores all over the country trying to get their hands on it.
The toy was simple. It was a stuffed Elmo — of Sesame Street fame — with a laugh box inside. So when kids “tickled” it, the toy would laugh like Elmo. The toy was an instant success and led to many versions released in the years since.
We’re all hoping for similar success when we release a product or feature. Take something that already exists and add new technology to it. Voila! It should be a hit, right?
It’s not so easy. Just because Tyco hit it big with Tickle Me Elmo, doesn’t mean adding AI to your product will bring similar success. Sure, your product isn’t a toy, but your users might not take it seriously if the AI features you add miss the mark. And then, it could end up doing as well as Mattel’s Happy Family Midge.
When you are adding AI to your product, you should follow a structured approach so that the AI is fully aligned with your product’s goals and its user experience. Here are steps you should take as you implement AI in SaaS products.
6 steps to implement AI in B2B SaaS products
Define business goals
Research user needs
I always recommend starting with users when you decide to implement AI in SaaS products.
Find out their pain points and where AI can help. This requires gathering insights from your users, stakeholders, and competitors. We typically gather insights through qualitative user interviews. Surveys and usability testing can also help you understand customer needs and expectations.
Design the feature
Really think about how users would interact with the AI feature. This should not be done in isolation. Cross-functional teams of product managers, data scientists, and UX or product designers should collaborate to define clear AI use cases.
It doesn’t matter what AI feature it is — personalizing user experiences, improving customer support, or optimizing workflows — make sure the AI solution aligns with your business objectives.
What it might look like
Let’s imagine that your business goal is to reduce customer churn. A B2B SaaS company might use AI to improve customer churn prediction. You would need to define what user behaviors indicate churn and how AI can predict it early enough for interventions.
Evaluate your data
Research
Before you move any further, you should conduct a data audit in order to ensure your product has enough, high-quality data for training AI models. AI’s success heavily relies on the quantity and quality of data, so it’s essential to clean, structure, and label your data so the algorithm can learn from it. This is where a well-designed information architecture comes in.
Design workflows
Working with data scientists, design workflows so you can collect data and then clean it up. In addition, include mechanisms in the UX design to capture more relevant data from users, so you can make sure the product has the necessary data points to make accurate predictions.
What it might look like
Let’s imagine you want to implement personalized dashboards. Take steps to ensure that your SaaS product tracks relevant user data, like interaction frequency, feature usage, and support requests.
Build and train AI models
Research through experimentation
After data evaluation, work with your data science team to build machine learning models that solve the defined use cases. This step requires experimenting with different algorithms and testing them on historical data.
Design the overall experience
Think about how AI will be part of the user experience. Will it work in the background? Will it need to interact with users? Design interfaces that present AI-generated insights in a clear, non-technical way for end users.
What it might look like
Spotify created its recommendation system to continually learn from user behavior and integrate seamlessly into the UI with simple and intuitive playlist suggestions.
Prototype and iterate
Research through testing
Before launching a full AI solution, develop a prototype or minimum viable product (MVP) to test the AI’s performance on a small scale. Gather feedback through user testing to determine whether the AI meets expectations and solves real user problems.
Design the journey
Create wireframes and prototypes that demonstrate how the AI feature will fit into the user journey. Involve users in testing the prototypes so that you can make sure the AI elements enhance the user experience without creating confusion or friction.
What it might look like
When implementing an AI-driven customer success feature, test how users respond to automated suggestions for product tutorials or resources before scaling up the deployment.
Implement AI with transparency and control
The research on trust
Research shows that users are more likely to trust AI when they understand how it works. As you implement AI in SaaS products, gather insights on users’ expectations around AI transparency and control. For example, do they want to know how AI-driven decisions are made, or do they prefer automated processes without much explanation?
Design with transparency in mind
Ensure that your AI feature offers transparency by providing explanations or insights into how AI-generated recommendations or decisions were made. Additionally, give users control over how AI interacts with them, such as the ability to opt-out of certain AI features or adjust personalization settings.
What it might look like
Google’s smart reply feature in Gmail gives users the option to use or ignore AI-generated email replies, maintaining control over their interactions with AI.
Monitor, optimize, and scale
Research through ongoing metrics
Finally, it’s important to continuously monitor the performance of the AI models after they go live. Use metrics to follow user engagement, prediction accuracy, and overall user satisfaction.
These will help you determine the success of the feature. You can also conduct periodic user interviews to make sure that the AI features remain relevant and valuable as user needs evolve.
Iterate the design
Build in iterative design processes that allow for ongoing optimization of the AI features. Design updates should be data-driven, and improvements to AI functionality should be seamlessly integrated into the product.
What it might look like
After rolling out an AI-powered churn predictor, a SaaS company can track the feature’s effectiveness in preventing churn and refine the model based on real-time user feedback and engagement data.
Conclusion: Align AI with business and user needs
When you implement AI in SaaS products requires thoughtful planning from research to design. Ensure that AI features are clearly aligned with business goals, well-supported by clean and relevant data, and designed to enhance the user experience. Transparency, iteration, and user feedback are key to building trust and maximizing the value AI brings to both the product and its users.
Including these practical steps in your article will provide product leaders with a clear roadmap for integrating AI successfully.