A step-by-step guide to using AI to solve common UX challenges in enterprise systems and boost user productivity
Enterprise systems have always been notorious for their complexity. They serve a wide range of users—each with unique needs—and often come with sprawling workflows and outdated interfaces. It’s no surprise that user experience (UX) in enterprise applications can feel like an afterthought, leaving employees frustrated and productivity stagnant.
Enter artificial intelligence (AI), a transformative tool that’s revolutionizing enterprise systems. AI in enterprise UX is changing the game, offering solutions that eliminate inefficiencies and make these tools as intuitive and user-friendly as consumer-facing applications. From automating tedious tasks to delivering personalized experiences, it’s a powerful way to modernize outdated systems.
So, how can product leaders integrate AI in enterprise UX effectively? This guide outlines a clear, step-by-step approach to harness its potential and elevate your enterprise software.
In this article
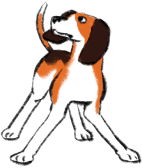
Why AI in enterprise UX matters
Before we dive into the how, let’s address the why. Why is AI in enterprise UX tools such a big deal?
The reality is that enterprise users expect their work tools to perform like the apps they use in their personal lives. They want speed, simplicity, and systems that “just work.” AI delivers on these expectations by tackling common pain points in enterprise UX, such as:
- Complex navigation: AI-powered search tools use natural language processing (NLP) to interpret user intent and surface relevant information.
- Repetitive tasks: Machine learning algorithms can automate routine workflows, freeing up users for higher-value activities.
- Personalization gaps: AI adapts interfaces to individual user roles, showing the most relevant features and information.
When done right, integrating AI into enterprise systems doesn’t just improve usability. It drives business outcomes like increased productivity, reduced training time, and happier employees.
How to integrate AI into enterprise UX systems: Step-by-step guide

Step 1: Identify UX pain points where AI can help
Integration begins with understanding your system’s challenges and opportunities. A thoughtful approach starts with a comprehensive UX audit of your enterprise system to uncover where users struggle most. This involves collecting quantitative data, such as error rates or time-on-task metrics, alongside qualitative insights from user feedback and observations. By taking the time to map out the friction points, you can make targeted decisions about where AI can provide the most value.
Here are three common areas where AI in enterprise UX can make a significant impact:
1. High-friction workflows
Many enterprise tools require users to perform multiple unnecessary steps to complete even simple tasks. AI can streamline these processes by identifying patterns in user behavior and offering predictive suggestions. For example:
- AI can recommend commonly used actions based on previous activity, reducing the time spent navigating menus.
- Intelligent assistants can guide users step-by-step through complex processes, such as completing compliance forms or scheduling recurring tasks.
2. Information bottlenecks
Enterprise systems are notorious for burying critical insights in layers of menus or across disparate platforms. AI-powered tools can resolve these issues through enhanced search functionality and contextual recommendations.
- AI-powered search engines: Tools using natural language processing (NLP) can interpret user queries in plain language and surface the most relevant results.
- Context-aware dashboards: AI can prioritize displaying the data users need most based on their roles and historical interactions.
Example: If your internal knowledge base requires users to sift through endless categories to find a procedure, implementing AI search can drastically improve efficiency by predicting the intent behind search queries and learning from user feedback.
3. Training challenges
New employees often face steep learning curves when navigating enterprise systems. Training can be costly and time-consuming, particularly for tools with outdated or overly complex interfaces. AI can simplify onboarding through:
- Interactive tutorials: AI systems that adapt to the pace of individual users can guide new employees in real time.
- Personalized learning paths: AI can recommend training modules tailored to each user’s role and experience level.
- Automated error correction: AI can proactively identify mistakes and suggest corrections, reducing frustration for less experienced users.
How this might work
Imagine an HR system where users struggle to find policy documents or manage onboarding workflows. By conducting a UX audit, you might discover that employees spend significant time searching for forms. Introducing an AI-powered assistant to guide users directly to their required documents or automate the onboarding checklist can save hours of work and increase satisfaction.
Actionable tip: To effectively identify pain points, involve both your end users and your support team. End users provide firsthand insights into frustrations, while support staff can highlight recurring complaints and technical obstacles. Mapping these issues to specific AI capabilities ensures a strategic and focused implementation.
By addressing these pain points with AI in enterprise UX, you create systems that not only meet user needs but also drive efficiency and productivity across the board.
Step 2: Define clear goals for AI integration
With the pain points identified, the next step is to set clear, measurable goals for integrating AI into your enterprise UX. Without defined objectives, AI projects risk becoming experiments that fail to deliver tangible value. Your goals should bridge the gap between user needs and business priorities, ensuring AI investments align with your organization’s broader strategy.
Here’s how to set meaningful goals for AI integration:
1. Clarify desired outcomes
Start by asking, What are we trying to achieve? Consider the specific challenges uncovered in Step 1 and how AI could address them. Your outcomes should focus on both improving the user experience and driving business benefits. Examples include:
- Improving efficiency: Reducing time spent on repetitive or manual tasks.
- Boosting accuracy: Increasing the precision of searches, recommendations, or workflow outputs.
- Enhancing usability: Making systems more intuitive for diverse user roles.
Example: If users struggle to complete complex workflows due to redundant steps, your desired outcome might be to reduce task completion time by 30 percent. Similarly, if employees often miss critical updates buried in dashboards, you might aim to increase engagement with key insights by 50 percent.
2. Make goals measurable
Vague goals like “improve usability” or “make workflows faster” aren’t actionable. Instead, focus on measurable key performance indicators (KPIs) that demonstrate success.
Here are some sample metrics for AI in enterprise UX:
- Time-on-task: Measure the time it takes for users to complete specific tasks before and after AI integration.
- Error reduction: Track the number of user errors in workflows and how AI tools help prevent them.
- Adoption rates: Monitor how quickly users adopt new AI-driven features compared to traditional workflows.
- Satisfaction scores: Use post-interaction surveys to assess user perceptions of AI tools.
Example: If your knowledge base search currently takes users an average of 5 minutes to find a document, set a measurable goal to reduce that time by 60 percent with AI-powered search enhancements.
3. Align goals with business objectives
AI integration should deliver results that resonate not only with users but also with organizational stakeholders. Link UX goals to larger business priorities, such as:
- Cost savings: Reducing the time employees spend on inefficient processes directly cuts operational costs.
- Revenue growth: Faster workflows or better usability can help sales teams close deals more efficiently.
- Employee retention: Intuitive, user-friendly tools reduce frustration and foster higher job satisfaction.
Example: If your sales team struggles with clunky CRM tools, improving UX with AI-driven dashboards could lead to faster deal cycles, contributing to overall revenue growth.
4. Set short- and long-term targets
Some AI benefits are immediate, while others require time to develop. Create a roadmap with short- and long-term milestones to maintain momentum and demonstrate progress to stakeholders.
- Short-term targets:
- Automate one repetitive workflow within three months.
- Improve search accuracy for a single department by 20% in the first phase.
- Long-term goals:
- Scale AI-powered features to the entire organization within two years.
- Reduce system-wide user error rates by 50% over 18 months.
Breaking down large goals into smaller, achievable steps keeps your AI integration on track and allows you to iterate based on early results.
5. Communicate Goals Across Teams
Once goals are defined, ensure they are communicated clearly across all involved teams. Collaboration between UX designers, developers, product managers, and data scientists is essential for success. By sharing a unified vision of the outcomes you aim to achieve, you can align everyone’s efforts and minimize silos.
Example: If the goal is to reduce task completion time, developers need to understand how AI algorithms will work in the system, while UX designers need to ensure interfaces are intuitive for users interacting with those algorithms.
Why defining goals matters
Setting clear goals provides focus and accountability. It ensures that your AI integration efforts stay grounded in real user needs and deliver measurable improvements. Without these benchmarks, it’s easy for AI projects to veer off course, resulting in tools that look impressive on paper but fail to add meaningful value in practice.
By starting with defined objectives, you lay the foundation for successful implementation, delivering outcomes that improve enterprise UX and advance organizational priorities.
Pro Tip: Use goals to guide continuous evaluation. As AI tools are rolled out, revisit your initial objectives to measure progress and refine your strategy. Flexibility is key—your goals may evolve as new user insights emerge.
Step 3: Choose the right AI technologies
Selecting the right AI technologies for enterprise UX can make or break your integration strategy. With countless tools and platforms available, it’s essential to choose solutions that align with your organization’s specific pain points, goals, and infrastructure. Not all AI is created equal—what works for one enterprise might not work for another. Here’s how to make an informed decision and leverage the most effective technologies for AI in enterprise UX.
Start with user-centric goals
The first step in choosing AI technologies is tying them directly to user needs and business objectives. Ask:
- What problem is this technology solving?
- How will it improve the user experience?
- Does it integrate seamlessly into existing workflows?
For example, if users struggle with locating documents or data, Natural Language Processing (NLP) is likely the best fit. If your challenge involves automating repetitive, high-volume tasks, Machine Learning (ML) may be more appropriate. Always let the problem guide the solution—not the other way around.
Popular AI technologies for enterprise UX systems
1. Natural Language Processing (NLP)
NLP is a game-changer for search and communication features in enterprise systems. It enables users to interact with your software using natural, conversational language, making complex systems easier to navigate.
Use cases:
- Smart search tools that understand intent and context (e.g., “Find the latest expense report”).
- Chat interfaces where users can ask questions and receive relevant answers without diving into documentation.
- Multilingual support for global enterprises, where NLP can translate queries and responses in real time.
Example: A financial firm implemented NLP to improve search functionality in its compliance portal. Instead of manually filtering through regulations, users now ask plain-language questions like, “What’s the reporting deadline for Q2?” and receive instant, accurate results.
2. Machine Learning (ML)
ML allows systems to analyze data, learn patterns, and make decisions without explicit programming. This is ideal for creating proactive, predictive, and adaptive user experiences.
Use cases:
- Predictive analytics: Recommending relevant actions or data based on past behavior.
- Automation: Streamlining repetitive tasks like expense approvals or inventory updates.
- Intelligent workflows: Helping users identify the next best step in a process.
Example: In a logistics company, ML-powered dashboards predict inventory shortages based on historical trends and seasonal patterns, helping employees act before disruptions occur.
3. AI chatbots
Chatbots provide real-time assistance, reducing dependency on help desks or support teams. Modern chatbots use conversational AI to engage with users more naturally and solve issues efficiently.
Use cases:
- Employee self-service: Assisting with tasks like resetting passwords or accessing HR forms.
- Workflow support: Guiding users through multi-step processes, such as onboarding a new vendor.
- FAQ management: Answering common user queries, saving time for support teams.
Example: A manufacturing enterprise deployed an AI chatbot to assist factory managers with equipment maintenance schedules. Instead of searching through manuals, users ask the chatbot for maintenance steps or troubleshooting tips.
4. Computer vision
Computer vision enables machines to interpret and analyze visual data, making it ideal for specific industries like healthcare, manufacturing, and construction.
Use cases:
- Quality control in manufacturing: Identifying defects or irregularities in products
- Document processing: Automatically extracting data from forms or invoices
- Visual data analytics: Recognizing patterns in medical imaging or satellite photos
Example: A healthcare provider uses computer vision to process insurance claims, scanning documents to extract and validate relevant data automatically.
Third-party tools vs. custom solutions
Once you’ve identified the right technology, decide whether to use off-the-shelf tools, build custom solutions, or adopt a hybrid approach. Each option has its pros and cons:
1. Third-party AI tools
- Pros: Quick deployment, lower upfront costs, and pre-built integrations.
- Cons: Limited customization and potential compatibility issues with existing systems.
Example: An enterprise HR platform integrates a third-party chatbot tool to provide instant answers to employee questions about benefits, saving time for the HR team.
2. Custom-built AI solutions
- Pros: Tailored to your organization’s unique needs and workflows, with greater control over functionality.
- Cons: Higher costs and longer development timelines.
Example: A logistics company develops a custom ML model to predict delays in shipments based on weather, traffic, and historical data.
3. Hybrid approach
- Combine third-party tools with custom integrations to get the best of both worlds.
Example: A retailer uses a third-party NLP engine for search functionality but customizes the interface to match its branding and user workflows.
Evaluation criteria for AI technologies
To ensure you select the right technology, evaluate potential solutions against these criteria:
- Scalability: Can the technology grow with your organization and handle increasing data or user demands?
- Integration: How easily does it fit into your existing systems and workflows?
- User Experience: Is the AI intuitive and easy to use for both end users and administrators?
- Data Security: Does it comply with your organization’s data privacy and security standards?
- Support and Maintenance: What resources are available for troubleshooting, updates, and training?
Pro tip: Don’t get caught up in the latest AI trends or buzzwords. Focus on practical solutions that address real user needs and align with your organization’s goals.
Selecting the right AI technologies for enterprise UX is about finding tools that solve specific problems, integrate seamlessly into existing systems, and deliver measurable value. By making thoughtful, user-focused choices, you can set your AI integration project up for success.
Step 4: Collaborate with cross-functional teams
AI integration isn’t a solo project. It requires collaboration across multiple teams:
- UX designers to ensure AI features align with user needs.
- Data scientists to build, train, and optimize AI models.
- Developers to integrate AI capabilities into your existing systems.
- Product managers to keep the effort aligned with strategic goals.
Hold workshops to align everyone on a shared vision for AI in enterprise UX, and establish clear roles and responsibilities for each team.
Step 5: Build AI-driven features incrementally
Start small. Instead of overhauling your entire system, focus on one or two high-impact areas where AI can make an immediate difference. For example:
- Introduce an AI-powered search tool to simplify document retrieval.
- Implement automated workflow suggestions for routine tasks like expense reporting.
This incremental approach allows you to test features, gather feedback, and refine your solutions before scaling them across the organization.
Step 6: Test and iterate continuously
AI thrives on iteration. Once you’ve launched your first set of features, gather data to assess their impact. Key metrics might include:
- Task completion times
- User satisfaction scores
- Error rates or failed interactions
Conduct usability testing with real users to identify areas for improvement. Remember: AI is only as good as the data and feedback it receives, so keep optimizing.
Challenges of integrating AI into enterprise UX systems
While the potential is immense, integrating AI in enterprise UX isn’t without its challenges. Common roadblocks include:
- Data quality issues: AI relies on clean, well-structured data. Without it, your AI models might produce unreliable results.
- Legacy systems: Many enterprise applications weren’t designed with AI in mind, making integration tricky.
- Skill gaps: Not every organization has in-house expertise in AI development.
Solutions:
- Invest in data cleaning and preparation to ensure AI models perform well.
- Use middleware or APIs to bridge gaps between AI tools and legacy systems.
- Partner with external experts to fill skill gaps while upskilling your internal team.
Real-world examples of AI in enterprise UX
Companies worldwide are implementing AI to solve real challenges and elevate their enterprise systems. Here are actual examples of how AI is driving innovation across industries.
1. Salesforce Einstein: Smarter CRM tools
Salesforce Einstein uses AI to revolutionize CRM systems by analyzing customer data and providing actionable insights. It automates lead scoring, prioritizing the most likely conversions, and recommends follow-up actions for sales teams.
Impact:
- Sales teams spend less time analyzing data and more time closing deals.
- Businesses report increased customer engagement through personalized recommendations.
2. Holcim: Predictive maintenance in manufacturing
Holcim, a global leader in building materials, has implemented AI-based machine intelligence across over 100 plants. The system predicts equipment failures by analyzing operational data, enabling preemptive maintenance that reduces downtime.
Impact:
- Prevented unplanned downtime, significantly improving operational efficiency.
- Reduced maintenance costs by addressing issues before failures occur.
3. IBM Watson: AI-powered knowledge management
IBM Watson is used to enhance enterprise search capabilities, making internal knowledge bases more efficient. By employing natural language processing (NLP), Watson enables employees to ask questions in plain language and receive accurate, context-aware answers.
Example:
An insurance company integrated Watson to help employees find policy information quickly, cutting down search times and improving service quality.
Impact:
- Faster information retrieval led to a 50 percent improvement in employee productivity.
- Improved employee satisfaction by eliminating the frustration of manual searches.
4. LinkedIn’s AI-driven onboarding system
LinkedIn uses AI to guide new hires through onboarding, helping them complete paperwork, set up accounts, and learn about company policies. The AI assistant answers common questions in real-time, reducing HR’s workload.
Impact:
- Onboarding time dropped by 40 percent, enabling faster integration of new hires.
- HR teams saved hours previously spent on repetitive tasks.
5. Siemens: AI-powered ERP dashboards
Siemens implemented AI-driven dashboards within its ERP systems to tailor the user interface to specific roles. Supply chain managers see inventory updates, while finance teams have access to budget forecasting tools, all powered by machine learning.
Impact:
- Increased task completion rates as users accessed relevant data more easily.
- Reduced training time for new employees due to simplified interfaces.
6. Google AI: Visual data analysis for healthcare
Google’s AI algorithms are being used in healthcare for advanced pattern recognition in medical imaging. For example, its computer vision technology assists radiologists by detecting early signs of diseases like cancer with high accuracy.
Impact:
- Reduced diagnostic errors, improving patient outcomes.
- Enhanced efficiency by automating the analysis of thousands of medical images.
These examples showcase how organizations leverage AI in enterprise UX to address specific pain points, improve productivity, and enhance user satisfaction. Whether it’s smarter CRM tools, predictive maintenance, or role-specific dashboards, these real-world applications highlight the tangible benefits of implementing AI thoughtfully and strategically.
By learning from these successes, enterprise leaders can identify opportunities to integrate AI into their own systems and achieve meaningful results.
Conclusion
Integrating AI into enterprise UX isn’t just a tech trend — it’s a strategic move that can transform how your organization works. By addressing pain points, setting clear goals, and adopting a collaborative, iterative approach, product leaders can unlock the full potential of AI in enterprise UX.
Ready to explore how AI can improve your enterprise systems? Contact us for a free UX audit, and let’s identify opportunities to make your tools more intuitive, efficient, and user-friendly.